Biological age
Biological age was, in the past, thought to be synonymous of chronological age. However, we now know that while there is a strong association between both, biological age may significantly differ between individuals of identical chronological age, possibly due to reasons such as dissimilar health status.
The definition of chronological age is very straight forward: it is the number of years an individual has been alive. However, biological age is somewhat a more loosely defined concept. Biological age commonly refers to the physiological function and capability of an organism. It is a measure that aims to provide a proxy of physiological function and the degree of age-related traits in individuals. It can also be used to infer remaining years of life lived in good health (ie. remaining healthspan) and life expectancy (total remaining lifespan, including periods of poor health).
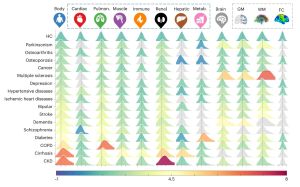
Organ clocks enabled elucidation of unique organ age profiles for chronic diseases and discovery of modifiable factors that can potentially lead to disease-specific longevity interventions targeted at specific body systems, ultimately extending lifespan.[1]
Several tools have been proposed to measure biological age. The most prominent is epigenetic clocks,[2] which provides information regarding the epigenetic status or DNA methylation marks of an individual, and which have shown to correlate with aging. Other aging clocks exist such as transcriptomics clocks,[3][4] glycation clocks,[5] telomere clocks,[6] microbiome clocks,[7][8] or more recently the DNAm PhenoAge,[9] which combines epigenetic marks and healthspan measurements.
Work by the Stroustrup's lab[10] has shown that C. elegans has at least two distinct biological ages, as interventions and mutations can decouple two aging phenotypes: remaining lifespan and time spent in vigorous movement. It is hypothesized that not only two, but multiple biological ages exist in worms, and by extrapolation even more in animals with higher physiological complexity: likely as many as components exist in the system. Therefore the lifespan of an organism might be more complex than it would be if dictated by a single aging process. Furthermore, it highlights the fact that simply because a measurement (such as epigenetic marks) correlates with lifespan, it does not necessarily provide a good aging biomarker, given that aging processes can be decoupled.
Importantly, currently available commercial kits that claim to measure biological age take samples from a single tissue (usually saliva or blood) and therefore provide information regarding the biological age of only that specific tissue. Future applications of biological clocks might be more accurate when integrating biological ages from multiple tissues.
See also
ExplaiNAble BioLogical Age (ENABL Age)
References
- ↑ 1.0 1.1 Tian, Y. E., Cropley, V., Maier, A. B., Lautenschlager, N. T., Breakspear, M., & Zalesky, A. (2023). Heterogeneous aging across multiple organ systems and prediction of chronic disease and mortality. Nature Medicine, 1-11. PMID: 37024597 DOI: 10.1038/s41591-023-02296-6
- ↑ Schumacher, A. (2009). An epigenetic clock: Anticorrelation & DNA methylation as biomarker for aging. https://doi.org/10.13140/RG.2.2.12457.83042
- ↑ Holzscheck, N., Falckenhayn, C., Söhle, J., Kristof, B., Siegner, R., & Werner, A. et al. (2021). Modeling transcriptomic age using knowledge-primed artificial neural networks. Npj Aging And Mechanisms Of Disease, 7(1). doi: 10.1038/s41514-021-00068-5
- ↑ Mamoshina, P., Volosnikova, M., Ozerov, I., Putin, E., Skibina, E., Cortese, F., & Zhavoronkov, A. (2018). Machine Learning on Human Muscle Transcriptomic Data for Biomarker Discovery and Tissue-Specific Drug Target Identification. Frontiers In Genetics, 9. doi: 10.3389/fgene.2018.00242
- ↑ Severin, F., Feniouk, B., & Skulachev, V. (2013). Advanced glycation of cellular proteins as a possible basic component of the “master biological clock”. Biochemistry (Moscow), 78(9), 1043-1047. doi: 10.1134/s0006297913090101
- ↑ Harley, C. (1991). Telomere loss: mitotic clock or genetic time bomb?. Mutation Research/Dnaging, 256(2-6), 271-282. doi: 10.1016/0921-8734(91)90018-7
- ↑ Galkin, F., Mamoshina, P., Aliper, A., Putin, E., Moskalev, V., Gladyshev, V., & Zhavoronkov, A. (2020). Human Gut Microbiome Aging Clock Based on Taxonomic Profiling and Deep Learning. Iscience, 23(6), 101199. doi: 10.1016/j.isci.2020.101199
- ↑ Gopu, V., Cai, Y., Krishnan, S., Rajagopal, S., Camacho, F., & Toma, R. et al. (2020). An accurate aging clock developed from the largest dataset of microbial and human gene expression reveals molecular mechanisms of aging. doi: 10.1101/2020.09.17.301887
- ↑ Levine, M., Lu, A., Quach, A., Chen, B., Assimes, T., & Bandinelli, S. et al. (2018). An epigenetic biomarker of aging for lifespan and healthspan. Aging, 10(4), 573-591. doi: 10.18632/aging.101414
- ↑ Oswal, N. et al. (2022) “A hierarchical process model links behavioral aging and lifespan in C. elegans,” PLOS Computational Biology, 18(9). Available at: https://doi.org/10.1371/journal.pcbi.1010415.