Epigenetic clock
Epigenetic clocks are based on an individuals's DNA methylation (DNAm) status, referred to as DNAm age or epigenetic age. They can be used to estimate chronological age and might potentially measure some aspects of biological age. Some studies argue that epigenetic clocks can predict all-cause mortality better than chronological age and other traditional risk factors.[1] However, there is currently no definitive evidence that epigenetic clocks can predict remaining lifespan and future health status at the individual level (but can be useful at the population level).
It is worth noting that epigenetic clocks have not offered a causal explanation of aging. They represent a tool to measure biological age status and, in a way, they show us what we already knew: there is a change in tissue composition over time across different cell types, with cells accumulating a number of hallmarks of aging, specially “inflammaging”.[2][3] Nonetheless, epigenetic clocks might be useful in providing a solid framework to test rejuvenating interventions, such as epigenetic reprogramming.[4]
A variety of other aging clocks exist based on parameters other than the methylation status. These include transcriptomics clocks,[5][6] glycation clocks,[7] telomere clocks,[8] microbiome clocks,[9][10] or more recently the DNAm PhenoAge,[11] which combines epigenetic clocks with several measurements of functional performance.
General purpose of epigenetic clocks
People vary significantly in how they age, with various factors leading to accelerated aging. Some examples include depression, stress, poverty, HIV/AIDs, diabetes, smoking, Down Syndrome, accelerated aging syndromes (e.g. progerias) and in childhood cancer survivors.[12][13][14][15][16][17][18][19][20][21][22] By measuring biological age, researchers could identify people who exhibit accelerated aging or vice versa slow aging.[23][24] This would determine who might benefit the most from an anti-aging drug, and perhaps be used as a surrogate marker for more quickly identifying if an aging intervention slows or even reverses aging.[25]
Quantifying biological age is considered important for longevity research, as running clinical trials over several decades to show whether human life has been extended is unrealistic. Instead, it might be more practical to use biological aging clocks to predict if a therapy is likely to extend healthspan and lifespan within a shorter timeframe.
Mechanism
The epigenetic clock works by measuring DNA methylation levels, i.e., the number and distribution of methyl groups attached to the DNA molecule. These ‘tags’ signal genes to be turned on or off.
Epigenetic clocks appear to measure a universal feature of aging across species. The same algorithm, based on the same set of biomarkers (DNAm) has been shown to strongly predict chronological age in hundreds of animals, including mice, bats, and humans. Notably, the residual or unexplained variance of epigenetic clocks (such as GrimAge) for prediction of chronological age appears to further capture biological age.[26] For GrimAge, this aspect is referred to as AgeAccelGrim, where the regression of DNA GrimAge on chronological age predicts whether biological age is greater or lesser than chronological age.[26] In other words, epigenetic clocks accurately predict one's age based on various DNAm biomarkers, but the error in prediction reflects the differences in rates of biological aging between individuals.
DNA methylation is the attachment of a methyl group to one of the “links” in the DNA strain (specifically, cytosine nucleotide). This does not affect the content of the DNA itself, but it does affect how it is read and used by the cell. This is one of the group of changes called epigenetics – changes in the organism's physical function which do not alter the DNA sequence itself, but can be inherited under certain conditions.
There have been a number of studies showing that as humans (and other mammals) age, patterns of methylation in their DNA change in certain ways.[27] The exact patterns of change are quite complex and not yet fully described, but broadly, two tendencies have been detected. First, the global level of methylation decreases, unequally in different tissues (for example, in mice, methylation levels decreased in the brain, heart, and spleen, but not in the lungs or liver). Secondly, the local methylation levels increase in certain locations: CpG islands (regions on a DNA strain where the sequence cytosine-guanine occurs with high frequency) and bivalent chromatin domain promoters (a promoter is a DNA sequence which initiates the transcription of the gene following it).
These changes can be used to estimate the biological age of the organism, and there are a number of approaches to achieving this measurement, the most common being the Horvath’s clock, developed by Horvath et al. in 2013.[28] They used publicly available datasets of methylation data collected on Illumina chips, and analyzed 21,369 CpG sites available on both 27k and 450k chips (the number referring to the total number of sites that the chip analyzes). The team then used a penalized regression model (elastic net regularization, which is essentially a linear combination of lasso and ridge regularization penalties, which thus drives the model to have both smaller coefficients and fewer of them) to identify 353 sites providing the most signals, of which 193 correlated with age positively, and the remaining 160 negatively. The clock then applies a calibration function to the weighted average of these 353 sites methylation levels to determine the biological age.
Methylation marker genes associated with aging
Regarding the definition of the markers, many candidate loci have been proposed, such as ELOVL2 (cg16867657),[29] EDARADD,[30][31] C1orf132 (cg10501210),[32] TRIM59, FHL2, KLF14, PDE4C, FHL2 (cg22454769), OTUD7A (cg04875128), CCDC102B (cg19283806),[33] ASPA, and PENK.[34][35][36]
ELOVL2
Elongase of very long chain fatty acids 2 (ELOVL2) represents a robust candidate gene as (i) its epigenetic variability is highly correlated with age predictions, (ii) it is included in most current age prediction models, and (iii) it does not show tissue-specificity, as observed for most of the epigenetic markers identified so far.[37][38][39] Functionally, Elovl2 plays an irreplaceable role in the synthesis of poly unsaturated fatty acids (PUFA)s, which are critical for a range of biological processes. Impaired Elovl2 function disturbs lipid synthesis with increased endoplasmic reticulum (ER) stress and mitochondrial dysfunction, leading to key aging phenotypes at both cellular and physiological level. Elovl2 deficiency induced a switch in metabolism from the tri-carboxylic acid cycle to glycolysis, an effect which produces more reactive oxidative species (ROS), causes oxidative stress in cells, tissues, and organs, and also act as a messenger for inflammatory responses. In addition, PUFAs are essential in the resolution of inflammation. In addition to that, there was a dramatic accumulation of fatty acids upon Elovl2 knockout, including arachidonic acid. As accumulation of arachidonic acid might also contribute to inflammation for its being used for Prostaglandin E2 (PGE2) generation, PGE2 may be involved in inflammation upon Elovl2 knockout.[40] The accumulation of free fatty acids in the ER would damage ER function, resulting in an increased incidence of unfolded or misfolded protein load and chronic ER stress.[41]
Discovery
Changes in methylation levels with aging have been observed for some time.[42] The first work using epigenetic changes as a basis for biological clocks was published in 2009 by Schumacher.[43] In 2013, the labs of Trey Ideker and Kang Zhang at the University of California, San Diego published the Hannum epigenetic clock, which consisted of 71 markers which accurately estimate age based on blood methylation levels.[44] In the same year, the first multi-tissue epigenetic clock was developed by Steve Horvath, a professor of human genetics and of biostatistics at UCLA.[45] Horvath’s clock allows the measurement of the age of different tissues of the same organism with the same clock, so it is the most widely used in aging research today.
Epigenetic clocks and aging
Retroelement-based epigenetic clocks
Emerging evidence suggests a correlation between aging, chronic diseases, and the reactivation of specific retroelements, primarily LINEs and HERV-K-derived retrovirus like particles (RVLPs). These findings highlight the potential of DNA methylation states of specific retroelements as reliable predictors of chronical and potentially biological aging, complementing existing epigenetic clocks and offering an additional mechanism to consider in epigenetic clock signals. This permits the construction of retroelement-based epigenetic clocks to support the hypothesis of dysregulation of endogenous retroelements as a potential contributor to the biological hallmarks of aging and suggest that therapeutic interventions modifying the epigenetic states of specific retroelements in the human genome could have beneficial effects against a root cause of aging and disease.[46][47]
It is not yet known whether epigenetic changes are a cause or consequence of other biological aging mechanisms. Epigenetic clocks have been used in some clinical trials of longevity drugs in an attempt to measure biological age. However, to enable its use as a surrogate marker, validation of various epigenetic clocks will require large-scale randomized clinical trials.
Several theories have been proposed, and are discussed below:
Link with Hallmarks of Aging
There is evidence that changed methylation patterns can be linked to some of the hallmarks of aging: loss of proteostasis, mitochondrial dysfunction, stem cell exhaustion, and immunosenescence.[48] Lu, Yuancheng, et al. were able to reverse age-induced loss of sight from glaucoma, and even regenerate a mechanically damaged eye nerve, by manipulating methylation patterns in mice.[49] They used three out of the four so-called 'Yamanaka factors', which are proteins necessary for reprogramming adult somatic cells back to pluripotent stem cells. Using the factors in live organisms for prolonged periods of time is known to cause cancer by boosting up cell division. But, with the exclusion of one of these factors (c-Myc), that is known to be oncogenic. The other three factors were kept active in mice for over a year without inducing any tumors.
The induction of these factors allowed the mice to regrow a mechanically damaged optic nerve. Normally, a mouse's optic nerve can regrow during early development, but then loses this ability a few days after birth. In this experiment, adult mice were able to re-obtain a similar regenerative ability and regained around half of their lost visual acuity.
Another result achieved using the Yamanaka factors was the restoration of the vision of healthy, middle-aged (one-year-old) mice. Before treatment, these mice scored worse than the younger mice on tests of visual acuity, but one month after treatment, they had similar results
Information Theory of Aging
Another theory, popularised by Professor David Sinclair, is that epigenetic changes might be the master regulator of aging - known as the information theory of aging.
Since DNA is identical in every somatic cell, each cell needs to “know” which genes to read in order to differentiate itself from a stem cell and perform its function. For example, a neuron cell only expresses (i.e. uses) genes relevant for being a neuron, and not a muscle cell or a skin cell. This is achieved through methylation and other epigenetic mechanisms.
The theory goes that aging is fundamentally caused by the accumulation of the effects of errors in this process, eventually causing a cell to stop functioning normally and either become cancerous or die.
Relevance for longevity research
For discussion see Fig. 2 from.[50]
Smoking
Research shows that smoking increases epigenetic age of buccal cells, airway cells, esophagus tissue, and lung tissue. Quitting smoking causes the epigenetic age acceleration in airway cells (but not in lung tissue) to revert to the level of non-smokers.[51]
Obesity
Obesity (defined as increased BMI) has been shown to correlate with increased epigenetic age in a number of tissues. For liver tissue, one study found an average increase of approximately 2.2 years of epigenetic age for each 10 BMI units.[52] There was no correlation for blood cells, however. Another study found an increase of approximately 2.3 years per 10 BMI points for visceral adipose tissue (visceral fat).[53]
Depression
Major depressive disorder (MDD) was also found to be associated with increased epigenetic age. One study found increased epigenetic age in blood cells associated with symptoms of MDD and childhood trauma scores.[54] They also analyzed brain cells (collected post mortem) and found that increased epigenetic age correlated with MDD symptoms.
Centenarians
Those who live past the age of 100 have reduced DNAm levels, with a pattern of methylation that appears to correlate less in neighboring cytosine-phosphate-guanine (CpG) sites of the DNA of newborns, which were more homogenous.[55]
Partial epigenetic reprogramming
YuanCheng Lu and colleagues were able to reverse loss of sight in age-related and glaucoma induced retinal ganglion cell loss, and even regenerate a mechanically damaged eye nerve. This was achieved by manipulating methylation patterns in mice, using partial epigenetic reprogramming delivered via viral gene therapy.[49] Epigenetic clocks in this study demonstrated an apparent reversal of epigenetic age in mice treated with epigenetic reprogramming.[49]
Other uses
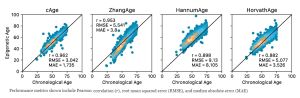
Epigenetic clock also has many other applications:
- Testing the validity of various theories of biological aging
- Diagnosing various age related diseases and for defining cancer subtypes
- Predicting/prognosticating the onset of various diseases
- Serving as surrogate markers for evaluating therapeutic interventions including rejuvenation approaches,
- Studying developmental biology and cell differentiation
- Forensic applications, e.g. to estimate the age of a suspect based on blood left at a crime scene
References
- ↑ Wertz, J., Caspi, A., Ambler, A., Broadbent, J., Hancox, R. J., Harrington, H., ... & Moffitt, T. E. (2021). Association of History of Psychopathology With Accelerated Aging at Midlife. JAMA psychiatry.
- ↑ Horvath, S. (2013). DNA methylation age of human tissues and cell types. Genome Biology, 14(10), 3156. https://doi.org/10.1186/gb-2013-14-10-r115
- ↑ López-Otín, C., Blasco, M., Partridge, L., Serrano, M., & Kroemer, G. (2013). The Hallmarks of Aging. Cell, 153(6), 1194-1217. doi: 10.1016/j.cell.2013.05.039
- ↑ Lu, Y., Brommer, B., Tian, X., Krishnan, A., Meer, M., & Wang, C. et al. (2020). Reprogramming to recover youthful epigenetic information and restore vision. Nature, 588(7836), 124-129. doi: 10.1038/s41586-020-2975-4
- ↑ Holzscheck, N., Falckenhayn, C., Söhle, J., Kristof, B., Siegner, R., & Werner, A. et al. (2021). Modeling transcriptomic age using knowledge-primed artificial neural networks. Npj Aging And Mechanisms Of Disease, 7(1). doi: 10.1038/s41514-021-00068-5
- ↑ Mamoshina, P., Volosnikova, M., Ozerov, I., Putin, E., Skibina, E., Cortese, F., & Zhavoronkov, A. (2018). Machine Learning on Human Muscle Transcriptomic Data for Biomarker Discovery and Tissue-Specific Drug Target Identification. Frontiers In Genetics, 9. doi: 10.3389/fgene.2018.00242
- ↑ Severin, F., Feniouk, B., & Skulachev, V. (2013). Advanced glycation of cellular proteins as a possible basic component of the “master biological clock”. Biochemistry (Moscow), 78(9), 1043-1047. doi: 10.1134/s0006297913090101
- ↑ Harley, C. (1991). Telomere loss: mitotic clock or genetic time bomb?. Mutation Research/Dnaging, 256(2-6), 271-282. doi: 10.1016/0921-8734(91)90018-7
- ↑ Galkin, F., Mamoshina, P., Aliper, A., Putin, E., Moskalev, V., Gladyshev, V., & Zhavoronkov, A. (2020). Human Gut Microbiome Aging Clock Based on Taxonomic Profiling and Deep Learning. Iscience, 23(6), 101199. doi: 10.1016/j.isci.2020.101199
- ↑ Gopu, V., Cai, Y., Krishnan, S., Rajagopal, S., Camacho, F., & Toma, R. et al. (2020). An accurate aging clock developed from the largest dataset of microbial and human gene expression reveals molecular mechanisms of aging. doi: 10.1101/2020.09.17.301887
- ↑ Levine, M., Lu, A., Quach, A., Chen, B., Assimes, T., & Bandinelli, S. et al. (2018). An epigenetic biomarker of aging for lifespan and healthspan. Aging, 10(4), 573-591. doi: 10.18632/aging.101414
- ↑ Wertz, J., Caspi, A., Ambler, A., Broadbent, J., Hancox, R. J., Harrington, H., ... & Moffitt, T. E. (2021). Association of History of Psychopathology With Accelerated Aging at Midlife. JAMA psychiatry.
- ↑ Bersani, F. S., Mellon, S. H., Reus, V. I., & Wolkowitz, O. M. (2019). Accelerated aging in serious mental disorders. Current opinion in psychiatry, 32(5), 381.
- ↑ Yegorov, Y. E., Poznyak, A. V., Nikiforov, N. G., Sobenin, I. A., & Orekhov, A. N. (2020). The link between chronic stress and accelerated aging. Biomedicines, 8(7), 198.
- ↑ Crimmins, E. M., Kim, J. K., & Seeman, T. E. (2009). Poverty and biological risk: the earlier “aging” of the poor. Journals of Gerontology Series A: Biomedical Sciences and Medical Sciences, 64(2), 286-292.
- ↑ [./Https://clinicalepigeneticsjournal.biomedcentral.com/articles/10.1186/s13148-019-0777-z Wu, X., Huang, Q., Javed, R., Zhong, J., Gao, H., & Liang, H. (2019). Effect of tobacco smoking on the epigenetic age of human respiratory organs. Clinical epigenetics, 11(1), 1-9.]
- ↑ Aung, H. L., Aghvinian, M., Gouse, H., Robbins, R. N., Brew, B. J., Mao, L., & Cysique, L. A. (2020). Is There Any Evidence of Premature, Accentuated and Accelerated Aging Effects on Neurocognition in People Living with HIV? A Systematic Review. AIDS and Behavior, 1-44.
- ↑ Aguayo-Mazzucato, C., Andle, J., Lee Jr, T. B., Midha, A., Talemal, L., Chipashvili, V., ... & Bonner-Weir, S. (2019). Acceleration of β cell aging determines diabetes and senolysis improves disease outcomes. Cell metabolism, 30(1), 129-142.
- ↑ Gensous, N., Bacalini, M. G., Franceschi, C., & Garagnani, P. (2020, July). Down syndrome, accelerated aging and immunosenescence. In Seminars in Immunopathology (pp. 1-11). Springer Berlin Heidelberg.
- ↑ Yamaga, M., Takemoto, M., Shoji, M., Sakamoto, K., Yamamoto, M., Ishikawa, T., ... & Yokote, K. (2017). Werner syndrome: a model for sarcopenia due to accelerated aging. Aging (Albany NY), 9(7), 1738.
- ↑ Guida, J. L., Agurs-Collins, T., Ahles, T. A., Campisi, J., Dale, W., Demark-Wahnefried, W., ... & Ness, K. K. (2020). Strategies to Prevent or Remediate Cancer and Treatment-Related Aging. JNCI: Journal of the National Cancer Institute
- ↑ Kohanski, R. A., Deeks, S. G., Gravekamp, C., Halter, J. B., High, K., Hurria, A., ... & Sierra, F. (2016). Reverse geroscience: how does exposure to early diseases accelerate the age‐related decline in health? Annals of the New York Academy of Sciences, 1386, 30-44
- ↑ Dec, E., Clement, J., Cheng, K., Church, G. M., Fossel, M. B., Rehkopf, D. H., ... & Horvath, S. (2023). Centenarian clocks: epigenetic clocks for validating claims of exceptional longevity. GeroScience, 1-19. PMID: 36964402 DOI: 10.1007/s11357-023-00731-7
- ↑ Daunay, A., Hardy, L., Bouyacoub, Y., Sahbatou, M., Touvier, M., Blanché, H., ... & How-Kit, A. (2022). Centenarians consistently present a younger epigenetic age than their chronological age with four epigenetic clocks based on a small number of CpG sites. Aging, 14(19), 7718-7733. PMID: 36202132 PMC9596211 DOI: 10.18632/aging.204316
- ↑ Ferrucci, L., Gonzalez-Freire, M., Fabbri, E., Simonsick, E., Tanaka, T., Moore, Z., Salimi, S., Sierra, F., & Cabo, R. de. (2020). Measuring biological aging in humans: A quest. Aging Cell, 19(2), e13080. https://doi.org/https://doi.org/10.1111/acel.13080
- ↑ Jump up to: 26.0 26.1 Lu, A. T., Quach, A., Wilson, J. G., Reiner, A. P., Aviv, A., Raj, K., Hou, L., Baccarelli, A. A., Li, Y., Stewart, J. D., Whitsel, E. A., Assimes, T. L., Ferrucci, L., & Horvath, S. (2019). DNA methylation GrimAge strongly predicts lifespan and healthspan. Aging, 11(2), 303–327. https://doi.org/10.18632/aging.101684
- ↑ Fransquet, P. D., Wrigglesworth, J., Woods, R. L., Ernst, M. E., & Ryan, J. (2019). The epigenetic clock as a predictor of disease and mortality risk: A systematic review and meta-analysis. Clinical Epigenetics, 11(1), 62. https://doi.org/10.1186/s13148-019-0656-7
- ↑ Bocklandt, S., Lin, W., Sehl, M. E., Sánchez, F. J., Sinsheimer, J. S., Horvath, S., & Vilain, E. (2011). Epigenetic predictor of age. PLOS ONE, 6(6), e14821. https://doi.org/10.1371/journal.pone.0014821
- ↑ Manco, L., & Dias, H. C. (2022). DNA methylation analysis of ELOVL2 gene using droplet digital PCR for age estimation purposes. Forensic Science International, 333, 111206. PMID 35131731 doi:10.1016/j.forsciint.2022.111206
- ↑ Ni, X. L., Yuan, H. P., Jiao, J., Wang, Z. P., Su, H. B., Lyu, Y., ... & Yang, Z. (2022). An epigenetic clock model for assessing the human biological age of healthy aging. Zhonghua yi xue za zhi, 102(2), 119-124. PMID 35012300 doi:10.3760/cma.j.cn112137-20210817-01862
- ↑ Daunay, A., Hardy, L. M., Bouyacoub, Y., Sahbatou, M., Touvier, M., Blanché, H., ... & How-Kit, A. (2022). Centenarians consistently present a younger epigenetic age than their chronological age with four epigenetic clocks based on a small number of CpG sites. Aging, 14(19), 7718—7733. PMID 36202132 doi:10.18632/aging.204316
- ↑ Spólnicka, M., Pośpiech, E., Pepłońska, B., Zbieć-Piekarska, R., Makowska, Ż., Pięta, A., ... & Branicki, W. (2018). DNA methylation in ELOVL2 and C1orf132 correctly predicted chronological age of individuals from three disease groups. International journal of legal medicine, 132(1), 1-11. PMID 28725932 PMC 5748441 doi:10.1007/s00414-017-1636-0
- ↑ Fleckhaus, J., & Schneider, P. M. (2020). Novel multiplex strategy for DNA methylation-based age prediction from small amounts of DNA via Pyrosequencing. Forensic Science International: Genetics, 44, 102189. PMID: 31648151 DOI: 10.1016/j.fsigen.2019.102189
- ↑ Fan, H., Xie, Q., Zhang, Z., Wang, J., Chen, X., & Qiu, P. (2022). Chronological age prediction: developmental evaluation of DNA methylation-based machine learning models. Frontiers in Bioengineering and Biotechnology, 9, 1462. PMID: 35141217 PMCID: PMC8819006 DOI: 10.3389/fbioe.2021.819991
- ↑ Varshavsky, M., Harari, G., Glaser, B., Dor, Y., Shemer, R., & Kaplan, T. (2023). Accurate age prediction from blood using of small set of DNA methylation sites and a cohort-based machine learning algorithm. bioRxiv, 2023-01. https://doi.org/10.1101/2023.01.20.524874
- ↑ Jung, S. E., Lim, S. M., Hong, S. R., Lee, E. H., Shin, K. J., & Lee, H. Y. (2019). DNA methylation of the ELOVL2, FHL2, KLF14, C1orf132/MIR29B2C, and TRIM59 genes for age prediction from blood, saliva, and buccal swab samples. Forensic Science International: Genetics, 38, 1-8. PMID: 30300865 DOI: 10.1016/j.fsigen.2018.09.010
- ↑ Slieker, R. C., Relton, C. L., Gaunt, T. R., Slagboom, P. E., & Heijmans, B. T. (2018). Age-related DNA methylation changes are tissue-specific with ELOVL2 promoter methylation as exception. Epigenetics & chromatin, 11, 1-11. PMID: 29848354 PMCID: PMC5975493 DOI: 10.1186/s13072-018-0191-3
- ↑ Paparazzo, E., Lagani, V., Geracitano, S., Citrigno, L., Aceto, M. A., Malvaso, A., ... & Montesanto, A. (2023). An ELOVL2-Based Epigenetic Clock for Forensic Age Prediction: A Systematic Review. International Journal of Molecular Sciences, 24(3), 2254. PMID: 36768576 PMCID: PMC9916975 DOI: 10.3390/ijms24032254
- ↑ Sukawutthiya, P., Sathirapatya, T., & Vongpaisarnsin, K. (2021). A minimal number CpGs of ELOVL2 gene for a chronological age estimation using pyrosequencing. Forensic Science International, 318, 110631. PMID: 33279766 DOI: 10.1016/j.forsciint.2020.110631
- ↑ Li, X., Wang, J., Wang, L., Gao, Y., Feng, G., Li, G., ... & Zhang, K. (2022). Lipid metabolism dysfunction induced by age-dependent DNA methylation accelerates aging. Signal Transduction and Targeted Therapy, 7(1), 162. PMID: 35610223 PMC9130224 DOI: 10.1038/s41392-022-00964-6
- ↑ Siddiqui, A. J., Jahan, S., Chaturvedi, S., Siddiqui, M. A., Alshahrani, M. M., Abdelgadir, A., ... & Adnan, M. (2023). Therapeutic Role of ELOVL in Neurological Diseases. ACS omega. 8(11), 9764–9774 PMID: 36969404 PMC10034982 DOI: 10.1021/acsomega.3c00056
- ↑ Romanov, G. A., & Vaniushin, B. F. (1980). Intragenomic specificity of DNA methylation in animals. Qualitative differences in tissues and changes in methylation of repeating sequences during aging, carcinogenesis and hormonal induction. [Article in Russian]. Molekuliarnaia Biologiia, 14(2), 357-368. PMID: 7383031
- ↑ Schumacher, A. (2009). An epigenetic clock: Anticorrelation & DNA methylation as biomarker for aging. https://doi.org/10.13140/RG.2.2.12457.83042
- ↑ Hannum, G., Guinney, J., Zhao, L., Zhang, L., Hughes, G., Sadda, S., Klotzle, B., Bibikova, M., Fan, J.-B., Gao, Y., Deconde, R., Chen, M., Rajapakse, I., Friend, S., Ideker, T., & Zhang, K. (2013). Genome-wide methylation profiles reveal quantitative views of human aging rates. Molecular Cell, 49(2), 359–367. https://doi.org/10.1016/j.molcel.2012.10.016
- ↑ Horvath, S. (2013). DNA methylation age of human tissues and cell types. Genome Biology, 14(10), 3156. https://doi.org/10.1186/gb-2013-14-10-r115
- ↑ Ndhlovu, L. C., Bendall, M. L., Dwaraka, V., Pang, A. P., Dopkins, N., Carreras, N., ... & Corley, M. J. (2024). Retro‐age: A unique epigenetic biomarker of aging captured by DNA methylation states of retroelements. Aging Cell, e14288. https://doi.org/10.1111/acel.14288
- ↑ Ndhlovu, L. C., Bendall, M. L., Dwaraka, V., Pang, A. P., Dopkins, N., Carreras, N., ... & Corley, M. J. (2023). Retroelement-Age Clocks: Epigenetic Age Captured by Human Endogenous Retrovirus and LINE-1 DNA methylation states. bioRxiv. PMID: 38106164 PMC10723416 DOI: 10.1101/2023.12.06.570422
- ↑ Jiang, S., & Guo, Y. (2020, July 8). Epigenetic clock: DNA methylation in aging [Review Article]. Stem Cells International. https://doi.org/https://doi.org/10.1155/2020/1047896
- ↑ Jump up to: 49.0 49.1 49.2 Lu, Y., Brommer, B., Tian, X., Krishnan, A., Meer, M., Wang, C., Vera, D. L., Zeng, Q., Yu, D., Bonkowski, M. S., Yang, J.-H., Zhou, S., Hoffmann, E. M., Karg, M. M., Schultz, M. B., Kane, A. E., Davidsohn, N., Korobkina, E., Chwalek, K., … Sinclair, D. A. (2020). Reprogramming to recover youthful epigenetic information and restore vision. Nature, 588(7836), 124–129. https://doi.org/10.1038/s41586-020-2975-4
- ↑ Noroozi, R., Rudnicka, J., Pisarek, A., Wysocka, B., Masny, A., Boroń, M., ... & Pośpiech, E. (2024). Analysis of epigenetic clocks links yoga, sleep, education, reduced meat intake, coffee, and a SOCS2 gene variant to slower epigenetic aging. GeroScience, 46(2), 2583-2604. PMID: 38103096 PMC10828238 DOI: 10.1007/s11357-023-01029-4
- ↑ Wu, X., Huang, Q., Javed, R., Zhong, J., Gao, H., & Liang, H. (2019). Effect of tobacco smoking on the epigenetic age of human respiratory organs. Clinical Epigenetics, 11(1), 183. https://doi.org/10.1186/s13148-019-0777-z
- ↑ Horvath, S., Erhart, W., Brosch, M., Ammerpohl, O., von Schönfels, W., Ahrens, M., Heits, N., Bell, J. T., Tsai, P.-C., Spector, T. D., Deloukas, P., Siebert, R., Sipos, B., Becker, T., Röcken, C., Schafmayer, C., & Hampe, J. (2014). Obesity accelerates epigenetic aging of human liver. Proceedings of the National Academy of Sciences, 111(43), 15538–15543. https://doi.org/10.1073/pnas.1412759111
- ↑ de Toro-Martín, J., Guénard, F., Tchernof, A., Hould, F.-S., Lebel, S., Julien, F., Marceau, S., & Vohl, M.-C. (2019). Body mass index is associated with epigenetic age acceleration in the visceral adipose tissue of subjects with severe obesity. Clinical Epigenetics, 11(1), 172. https://doi.org/10.1186/s13148-019-0754-6
- ↑ Han, L. K. M., Aghajani, M., Clark, S. L., Chan, R. F., Hattab, M. W., Shabalin, A. A., Zhao, M., Kumar, G., Xie, L. Y., Jansen, R., Milaneschi, Y., Dean, B., Aberg, K. A., van den Oord, E. J. C. G., & Penninx, B. W. J. H. (2018). Epigenetic aging in major depressive disorder. American Journal of Psychiatry, 175(8), 774–782. https://doi.org/10.1176/appi.ajp.2018.17060595
- ↑ Heyn, H., Li, N., Ferreira, H. J., Moran, S., Pisano, D. G., Gomez, A., Diez, J., Sanchez-Mut, J. V., Setien, F., Carmona, F. J., Puca, A. A., Sayols, S., Pujana, M. A., Serra-Musach, J., Iglesias-Platas, I., Formiga, F., Fernandez, A. F., Fraga, M. F., Heath, S. C., … Esteller, M. (2012). Distinct DNA methylomes of newborns and centenarians. Proceedings of the National Academy of Sciences, 109(26), 10522–10527. https://doi.org/10.1073/pnas.1120658109
- ↑ Bernabeu, E., McCartney, D. L., Gadd, D. A., Hillary, R. F., Lu, A. T., Murphy, L., ... & Marioni, R. E. (2023). Refining epigenetic prediction of chronological and biological age. Genome Med 15, 12 https://doi.org/10.1186/s13073-023-01161-y